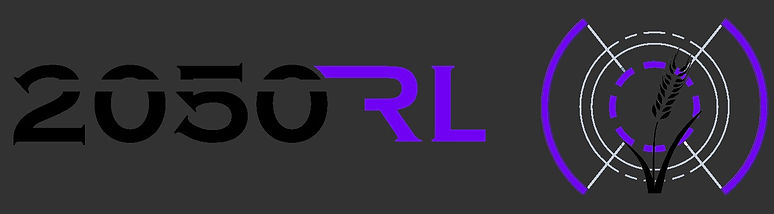
We aim to couple two modeling approaches – mechanistic and data driven models – to address shortcomings of both with respect to understanding and predicting toxin-producing cyanobacteria blooms. Currently, mechanistic understanding of cyanobacteria growth, bloom formation, and toxin production in response to a complex system of environmental and ecological drivers is lacking such that parameterizing models to represent these processes is challenging. Data-driven statistical models and machine learning approaches have, therefore, been favored in studies seeking to predict cyanobacterial blooms, cyanotoxins or other proxies under various environmental conditions. While these methods have had some success in predicting cyanoHABs in specific aquatic systems, they are limited in that they have not advanced understanding of the processes underlying toxic cyanoHABs and are limited in prediction to the range of environmental variables observed in the local datasets from which these models were created. We propose to integrate these disparate modeling paradigms by (1) overlaying data driven approaches to refine mechanistic model parameter calibration and (2) utilizing mechanistic models to validate machine learning frameworks. Because our proposed modeling framework is founded on mechanistic understanding, we aim to advance prediction of futures states that are not constrained to current conditions as well as to better understand changes that need to occur through watershed practices or in-lake interventions to achieve desired management targets.
Project Lead PI:Dr. Trisha Moore
PI: Dr. Aleksey Sheshukov
PI: Dr. Lior Shamir
PI: Dr. Dan Flippo